The Evolution of Machine Learning and Artificial Intelligence
The fields of Artificial Intelligence (AI) and Machine Learning (ML) have grown from theoretical concepts into fundamental technologies driving modern innovation. Below, traces the history of these fields, from their early philosophical beginnings to their current state as powerful tools reshaping industries.
Early Philosophical and Mathematical Roots
The roots of AI and ML trace back to ancient philosophy, where thinkers like Aristotle explored the nature of knowledge, reasoning, and the human mind. These early discussions laid the groundwork for formal logic, a key component in the development of AI.
In the 17th and 18th centuries, philosophers such as René Descartes and Gottfried Wilhelm Leibniz further advanced ideas about the mechanization of thought. Leibniz’s work on binary systems, in particular, provided a mathematical framework that would later influence the development of computing and AI.
The Dawn of Computing: 1930s-1940s
The modern era of AI began with the advent of digital computing in the mid-20th century. Alan Turing, often regarded as the father of computer science, introduced the concept of a universal machine (later known as the Turing Machine) in 1936. His work established the theoretical foundation for computers that could perform any calculation that could be described algorithmically.
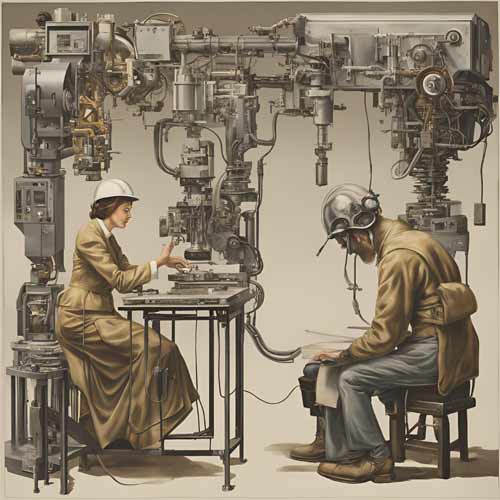
In the 1940s, the construction of the first digital computers, such as ENIAC and Colossus, marked a significant technological leap. These early machines were limited to specific tasks, but they demonstrated the potential for automated computation.
The Birth of Artificial Intelligence: 1950s-1960s
The formal birth of AI as a field of study is often credited to the 1956 Dartmouth Conference, organized by John McCarthy, Marvin Minsky, Nathaniel Rochester, and Claude Shannon. The conference introduced the term “artificial intelligence” and set the stage for decades of research and development.
During this period, researchers made significant strides in symbolic AI, where machines were programmed with explicit rules to solve problems. Early AI programs, such as the Logic Theorist (1955) and the General Problem Solver (1957), were capable of proving mathematical theorems and solving puzzles. These programs, however, struggled with more complex tasks due to the limitations of rule-based systems.
The Rise of Machine Learning: 1970s-1980s
As the limitations of symbolic AI became apparent, researchers began exploring alternative approaches, leading to the emergence of machine learning. Unlike symbolic AI, which relies on explicit programming, ML focuses on building systems that can learn from data and improve over time.
One of the earliest ML algorithms was the perceptron, developed by Frank Rosenblatt in 1958. The perceptron was a simple neural network model capable of binary classification. Although its capabilities were limited, it laid the groundwork for future developments in neural networks.
During the 1970s and 1980s, the field of machine learning expanded to include various statistical methods, such as decision trees, Bayesian networks, and clustering algorithms. These techniques allowed computers to analyze data more effectively and make predictions based on patterns.
The AI Winter and Renewed Interest: 1980s-1990s
The 1970s and 1980s also saw the onset of the “AI Winter,” a period characterized by reduced funding and interest in AI research due to unmet expectations and the challenges of building truly intelligent systems. Despite this, some areas of AI continued to advance, particularly in expert systems, which used rule-based methods to replicate human decision-making in specific domains.
By the late 1980s and early 1990s, renewed interest in AI was sparked by advances in computing power and the development of more sophisticated algorithms. The emergence of backpropagation, a method for training multi-layer neural networks, reinvigorated research in deep learning, a subfield of machine learning.
The Data Explosion and Modern AI: 2000s-Present
The turn of the 21st century marked a new era for AI and machine learning, driven by the explosion of data and the availability of more powerful computational resources. The rise of the internet, social media, and mobile technology generated vast amounts of data, providing the fuel for machine learning algorithms.
In the mid-2000s, deep learning emerged as a dominant force in AI. Neural networks with many layers (hence the term “deep”) began outperforming traditional machine learning methods in tasks such as image and speech recognition. Breakthroughs like the development of convolutional neural networks (CNNs) and recurrent neural networks (RNNs) played a crucial role in these advancements.
Notable milestones during this period include Google’s AlphaGo defeating the world champion in the game of Go in 2016, demonstrating the power of deep reinforcement learning. AI systems also became integral to industries such as healthcare, finance, and entertainment, where they are used for tasks ranging from diagnosing diseases to recommending movies.
Ethical and Societal Implications
As AI and machine learning technologies have matured, concerns about their ethical and societal implications have grown. Issues such as bias in algorithms, job displacement due to automation, and the potential misuse of AI in surveillance and warfare have prompted calls for responsible AI development and regulation.
Organizations and governments around the world are now focusing on creating frameworks to ensure that AI is developed and deployed in ways that are fair, transparent, and beneficial to society.
The Future of AI and Machine Learning
The future of AI and machine learning is full of possibilities. Advances in areas such as natural language processing, computer vision, and autonomous systems continue to push the boundaries of what machines can achieve. At the same time, interdisciplinary research is exploring ways to make AI more explainable, ethical, and aligned with human values.
Quantum computing, neuromorphic computing, and other emerging technologies may also play a significant role in the next wave of AI innovation, potentially leading to machines that can perform tasks that are currently beyond our imagination.
From its philosophical origins to its current status as a transformative technology, the history of AI and machine learning is a testament to human ingenuity and the relentless pursuit of knowledge. As we look to the future, the challenge will be to harness the power of these technologies in ways that enhance human life while addressing the ethical and societal challenges they present. The journey of AI is far from over, and the next chapters promise to be as exciting and impactful as the ones that have come before.
Leave a Reply