Revolutionizing Vehicles and Modern Control
Autonomous systems, powered by advancements in Artificial Intelligence (AI), are reshaping industries and transforming how we interact with the world. From self-driving cars to intelligent robots and modern control systems, autonomous systems are enabling machines to perform tasks with minimal human intervention. AI plays a pivotal role in enabling these systems to learn, adapt, and make decisions in real-time. Below, delves into how AI powers autonomous systems in various domains, such as autonomous vehicles, robotics, and modern control systems, highlighting their applications, challenges, and future potential.
Autonomous Vehicles: The Future of Transportation
Autonomous vehicles (AVs), also known as self-driving cars, are perhaps the most well-known application of AI-driven autonomous systems. These vehicles use a combination of sensors, machine learning algorithms, and control systems to navigate roads, avoid obstacles, and make real-time decisions with minimal human input.
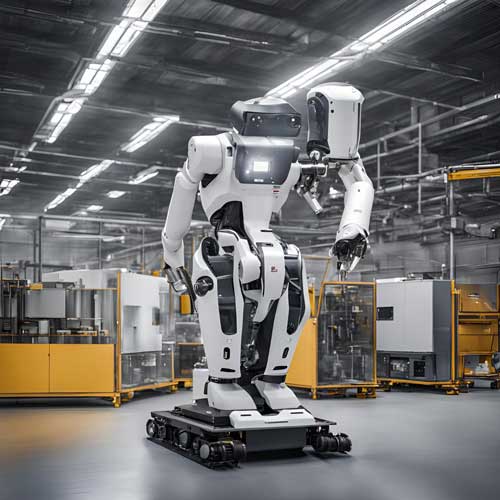
How AI Powers Autonomous Vehicles
Autonomous vehicles rely on AI to process vast amounts of data from sensors like LiDAR, radar, cameras, and GPS. AI-based algorithms help interpret this data to detect objects, track vehicles, predict pedestrian movements, and understand traffic rules. Key components of AI in autonomous vehicles include:
Perception: Using deep learning models like Convolutional Neural Networks (CNNs), AVs detect and classify objects such as other vehicles, pedestrians, road signs, and lane markings. Perception systems are crucial for enabling situational awareness.
Decision-Making: AI helps AVs make decisions such as when to change lanes, accelerate, brake, or turn. These decisions are based on data collected from the environment and predictions of potential hazards.
Path Planning: AI algorithms are used to plan safe and efficient routes for the vehicle to follow, taking into account traffic conditions, road layouts, and obstacles.
Control Systems: AI-based control systems ensure the vehicle maintains stability and handles complex maneuvers, such as parking or navigating sharp turns.
Levels of Autonomy in Vehicles
Autonomous driving is classified into five levels, with each level representing increasing degrees of autonomy:
Level 0: No automation; the human driver is responsible for all driving tasks.
Level 1: Driver assistance, where systems like adaptive cruise control assist but do not replace the driver.
Level 2: Partial automation, where the vehicle can steer, accelerate, and brake, but the driver must remain engaged.
Level 3: Conditional automation, where the vehicle handles most driving tasks, but the driver must intervene in certain situations.
Level 4: High automation, where the vehicle operates independently in specific conditions, but human intervention is still possible.
Level 5: Full automation, where the vehicle can operate independently in all conditions without human input.
Challenges in Autonomous Vehicles
Safety and Reliability: Ensuring that AVs operate safely in complex environments is a critical challenge. AI systems need to handle unpredictable road conditions, weather, and human behavior.
Data Privacy and Security: AVs rely on massive amounts of data to function. Ensuring the privacy and security of this data is essential to prevent hacking or misuse.
Regulation: Governments and regulatory bodies need to establish frameworks for testing, operating, and insuring autonomous vehicles on public roads.
Robotics: AI-Driven Machines in Action
Robotics has long been associated with automation, but AI has revolutionized the field, enabling robots to perform tasks that require perception, learning, and decision-making. Autonomous robots are being used across industries, from manufacturing and logistics to healthcare and agriculture.
AI in Robotics
AI enables robots to go beyond repetitive tasks by allowing them to adapt to new environments, learn from experiences, and collaborate with humans. Key AI components in robotics include:
Perception: Robots use AI to interpret sensory data from cameras, LiDAR, and other sensors to understand their surroundings. AI algorithms help them detect objects, navigate obstacles, and make sense of dynamic environments.
Autonomy and Decision-Making: Autonomous robots use reinforcement learning and decision-making algorithms to plan actions based on their current state and desired outcomes. These systems enable robots to perform tasks like assembling parts, sorting items, or navigating complex environments with minimal supervision.
Human-Robot Interaction: AI allows robots to interact naturally with humans, understanding speech, gestures, and facial expressions. Natural language processing (NLP) models enable robots to engage in meaningful conversations and respond to commands.
Applications of Autonomous Robots
Industrial Automation: AI-powered robots are transforming factories by automating repetitive tasks, such as assembly, welding, and quality control. These robots are highly efficient and reduce the need for human intervention in hazardous environments.
Healthcare: Autonomous robots assist in surgery, patient monitoring, and delivery of medications. AI-powered robotic surgery systems, like the da Vinci Surgical System, allow for precise and minimally invasive operations.
Logistics: Autonomous robots are revolutionizing logistics and warehousing operations by performing tasks such as picking, sorting, and transporting goods. Companies like Amazon and Ocado use AI-powered robots to optimize order fulfillment processes.
Agriculture: Autonomous robots equipped with AI can perform tasks such as planting, harvesting, and crop monitoring. They use machine vision and AI algorithms to detect weeds, pests, and disease, optimizing the use of resources.
Challenges in Robotics
Autonomous Navigation: Developing robots that can navigate complex, unstructured environments like cities or homes remains a significant challenge. AI systems must be able to handle uncertainty and adapt to dynamic changes in the environment.
Human-Robot Collaboration: Ensuring that robots can safely and effectively work alongside humans in shared spaces requires advanced AI systems that understand human behavior and intentions.
Modern Control Systems and Decision-Making
Autonomous systems extend beyond vehicles and robots to encompass a wide range of applications where intelligent decision-making and control are needed. AI is increasingly being used to optimize control systems in fields such as aerospace, energy, and manufacturing.
AI in Modern Control Systems
AI has revolutionized control systems by enabling more sophisticated decision-making and predictive capabilities. In traditional control systems, responses to inputs are based on predefined rules and models. However, AI-based control systems use machine learning to adapt dynamically to changing conditions, optimize performance, and predict future outcomes.
Predictive Maintenance: In industries like manufacturing and energy, AI-based control systems predict equipment failures before they occur. By analyzing sensor data, these systems can identify patterns that indicate potential issues, allowing for proactive maintenance.
Smart Grids: In the energy sector, AI-powered smart grids optimize the distribution of electricity based on real-time data from sensors and demand patterns. These systems can automatically balance supply and demand, improving efficiency and reducing costs.
Decision-Making with AI
Autonomous systems rely heavily on decision-making algorithms to handle complex scenarios, particularly when they encounter uncertainty or incomplete information. Techniques like reinforcement learning allow systems to learn optimal decision-making policies through trial and error. Decision-making AI is particularly critical in areas such as:
Autonomous Drones: Drones equipped with AI are used for applications like surveying, mapping, and delivering goods. These drones must make decisions on navigation, obstacle avoidance, and landing in real-time.
Financial Trading: Autonomous trading systems use AI to analyze market data and make decisions about buying and selling assets. These systems can respond to market conditions in milliseconds, outpacing human traders.
Challenges in Modern Control and Decision-Making
Scalability: Scaling AI-based control systems across large networks, such as national power grids or global supply chains, poses significant challenges due to the complexity and volume of data involved.
Trust and Explainability: Decision-making AI must be explainable to gain trust from users. As AI systems become more autonomous, ensuring that their decisions are transparent and interpretable is crucial for safety and accountability.
The Future of Autonomous Systems and AI
The future of autonomous systems is promising, with continued advancements in AI driving innovation across industries. Several trends are shaping the future of AI-driven autonomy:
Collaborative Autonomy
In the future, autonomous systems will collaborate seamlessly with each other and with humans. For instance, autonomous vehicles may work together to optimize traffic flow, while robots and humans will work side-by-side in smart factories.
Improved Learning Capabilities
Advances in machine learning, particularly in areas like transfer learning and meta-learning, will enable autonomous systems to learn faster, adapt to new tasks, and generalize across different environments.
Ethical and Societal Implications
As autonomous systems become more prevalent, ethical considerations surrounding safety, accountability, and job displacement will become increasingly important. Ensuring that these systems operate ethically and responsibly will be critical to gaining public trust.
Autonomous systems and AI are transforming industries, making machines more capable, adaptable, and efficient. From self-driving cars and intelligent robots to modern control systems, AI is at the heart of these innovations. While challenges remain—such as safety, scalability, and ethical considerations—the future of autonomous systems holds great promise, with AI continuing to push the boundaries of what machines can achieve.
Leave a Reply